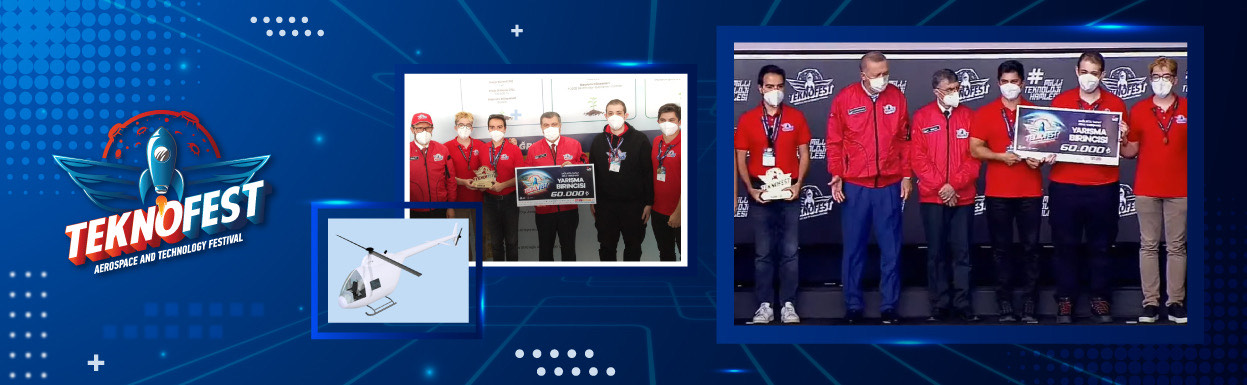
AI-DEO Teknofest category “Artificial Intelligence in Health Care”
Team Details
Dorukhan Afacan M.Sc
He graduated from the Department of Mecathronics Engineering, Sabancı University in 2015. He attended the University of Sheffield, where he pursued a Master’s degree in Computational Intelligence and Robotics and graduated with a degree. He has worked as a data scientist for 4 years in the fields of natural language processing, image processing, and predictive analytics. He took part in consultancy in the sector, R&D projects, national and international competitions related to these fields.
Askar Bozcan
He is a 4th year student in the CNG Program, METU NCC. He has perfected his skills in AI and Data Science and participated in a number of competitions. Below are some of the competitions he participated in;
He competed in the category “Artificial Intelligence” in Teknofest 2019 and won 3rd place.
He competed in a team contest in the category “Natural Language Processing” in Teknofest 2020 and his team was awarded 2nd place.
He competed in the competition Medathon (Artificial Intelligence in Health Care) and won 1st place.
Ertuğrul Demir
In 2019, he was graduated from Marmara University, where he pursued a degree in Business Administration.
He has been working as a data scientist for 6 months in the fields of image processing and natural language processing. He shares data at master level on the Kaggle platform. He was given a Google Developer Expert title for machine learning in March, 2021.
Onat Yapıcı
He is a 4th year student in Electrical-Electronics Engineering and Computer Engineering, Koç University. He has been engaged in data science for 2 years and conducting research in new generation image processing methods for 1 year.
Design Process
Problem Definition
A stroke is a health problem that occurs when the blood flow to the brain is restricted or cut off completely due to several reasons and that causes cell death. Today it appears to be one of the most common health problems that cause human death. A variety of modern techniques can be used to help health care professionals in the field of image processing to deal with this problem. By means of Convolutional Neural Networks (CNN), contrast-enhanced brain tomography scans of patients in question can be analyzed to obtain data to support the clinical decision support mechanisms
The competition has two parts/levels:
- Classification: Detection of the presence or absence of stroke in the given brain tomography scan
- Segmentation: Identification of the affected areas (bleeding or ischemia) if a stroke is detected.
Data Set
Approximately two thousand labeled, DICOM-format brain tomography scans were provided by the Ministry of Health, Republic of Turkey. A sample that represents the entire population of data cannot be made available, however, an image of a healthy brain sample, which is similar to the images in the given dataset, is provided below:
Modelling
‘Classification’ and ‘Segmentation’, as mentioned above, are the two tasks that need to be dealt with. Convolutional Neural Networks (CNN) are state of the art models most commonly applied to analyzing the two computer vision problems mentioned. However, the network structure has become more and more complex and varied; there are different CNN models with different structure of architectures. Therefore, different configurations were created for different CNN architectures to achieve the best outcomes.
The architectures used to solve the Classification problem are as follows:
- EfficientNet
- ResNeXt
- RegNety
Based on the experiments conducted, the model RegNety, which achieved the highest score, was selected to be used in the competition.
One model is usually not sufficient to solve the segmentation problem; a backbone model and a decoder model are also required. The backbone model extracts texts from images whereas the decoder model transforms the information collected by the backbone into a final segmentation map. (Within the framework of the competition, the segmentation map is responsible for visualizing the areas that were affected in the tomography mode.)
Therefore, combinations of different backbone and decoder models were used.
Backbone models are the same as those used for classification. The following backbone models were used:
- EfficientNet
- ResNeXt
- RegNety
However, decoder models are different. The decoder models used are as follows:
- UNet
- UNet++
- F
A combination of RegNety backbone and UNet++ decoder, which achieved the highest performance, was selected to be used in the competition based on the results of experiments conducted.
Competition Process
The competition consists of two rounds:
First Round
The competition proceeded in two phases. In the first round, each team was tasked with the detecting of presence or absence of stroke in the given brain tomography scan. In the brain scans provided, binary classification of stroke status (stroke present/absent) was performed. The results of applying a binary classifier to the dataset were evaluated by the referees by using the F1 score metric. Upon the completion of the first round, our team AI-DEO scored 0.9431 and won first place. Thus, our team became qualified for the second round.
Second Round
The competition proceeded with the second round focusing on the image segmentation problem. Each team was tasked with recognizing and scanning/marking the sites where the stroke is detected in brain scans with signs of stroke as well as classifying the type of stroke in the marked area correctly. The metric of success is calculated based on the ‘marked area’ and ‘correct area’ intersection and the accuracy of the classification model. The area is marked as ‘bleeding’ and ‘ischemia’. After the completion of the second round, our team AI-DEO won first place and we became the winner of the competition.
Second round; image demonstrating ischemic site:
(To protect patient privacy, the brain tomography scans provided by the Ministry of Health are not released).